Abstract—This paper presents a data-driven, Koopman operator-based modeling scheme for analyzing and predicting cross-coupling hysteresis effects of the piezoelectric tube scanners(PTSs) used in atomic force microscopes (AFMs). Such crosscoupling hysteresis effects between different PTS axes significantly reduce the positioning precision of AFMs. In contrast to most of the existing methods for PTS hysteresis, which involve complex nonlinear dynamics identification processes, the present study leverages the Koopman operator theory instead to treat the nonlinear hysteresis as a linear system. Therein, a Hankel extended dynamic mode decomposition (H-EDMD) algorithm is proposed to learn the finite-dimensional descriptions of the Koopman operator and the associated Koopman eigenspectrum. Moreover, the proposed H-EDMD even allows sparse sampling on the PTS systems, which is desirable in real industrial applications. Finally, extensive comparison experiments with a mainstream modified Prandtl-Ishlinskii model are conducted on an NTMDT Prima AFM to substantiate the effectiveness and superiority of the proposed H-EDMD method.
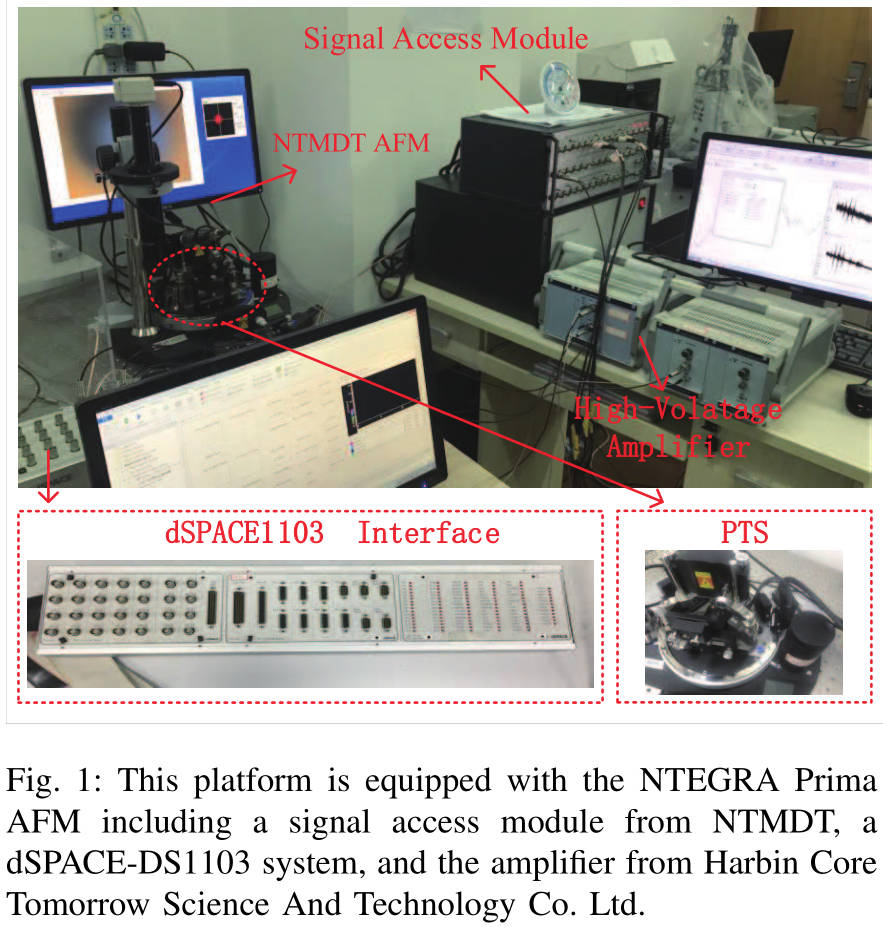
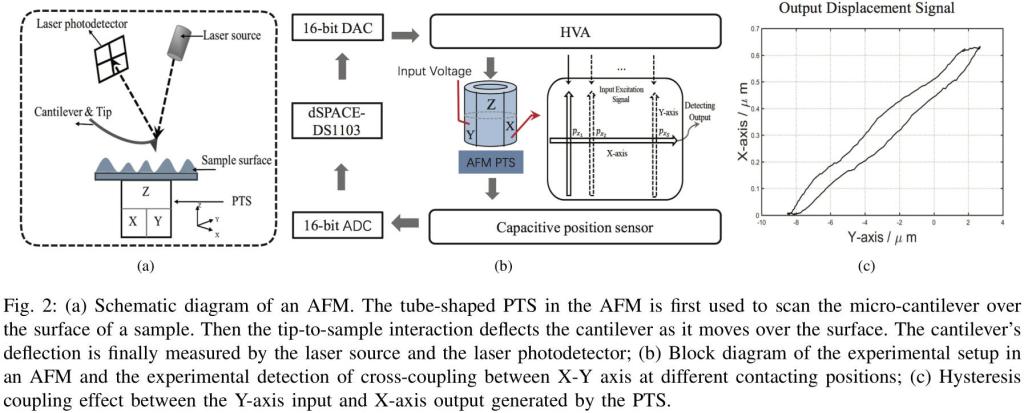
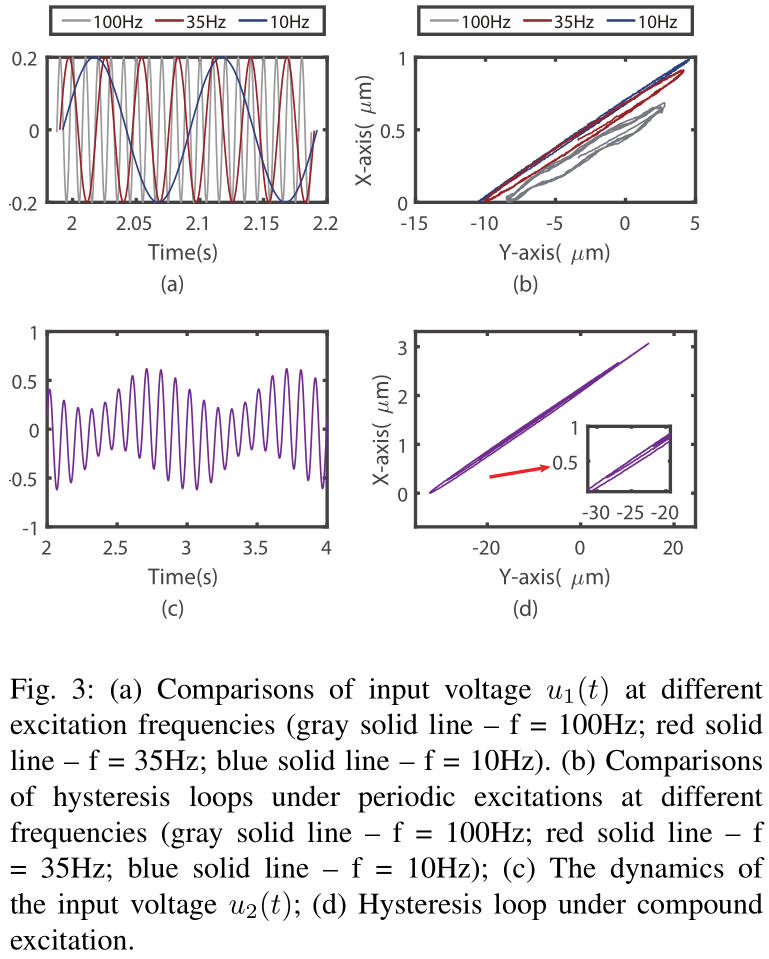
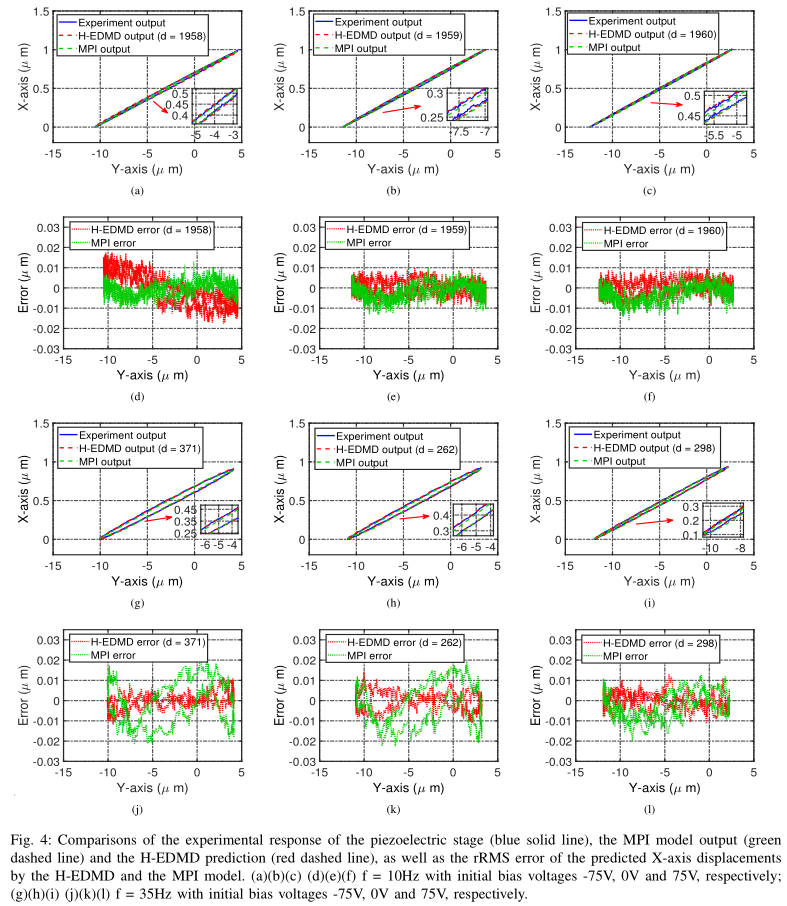