Abstract: Identifying structures of complex networks based on time series of nodal data is of considerable interest and significance in many fields of science and engineering. This paper presents a sparse Bayesian learning method for identifying structures of community-bridge networks, where nodes are grouped to form communities connected via bridges. By utilizing the structural information of such networks with unknown nodal dynamics and community formations, network structure identification is tackled similarly to sparse signal reconstruction with mixed sparsity mode. The proposed method is theoretically proved to be convergent. Its superiority to mainstream baselines is demonstrated via extensive experiments without the need for manual adjustment of regularization parameters.
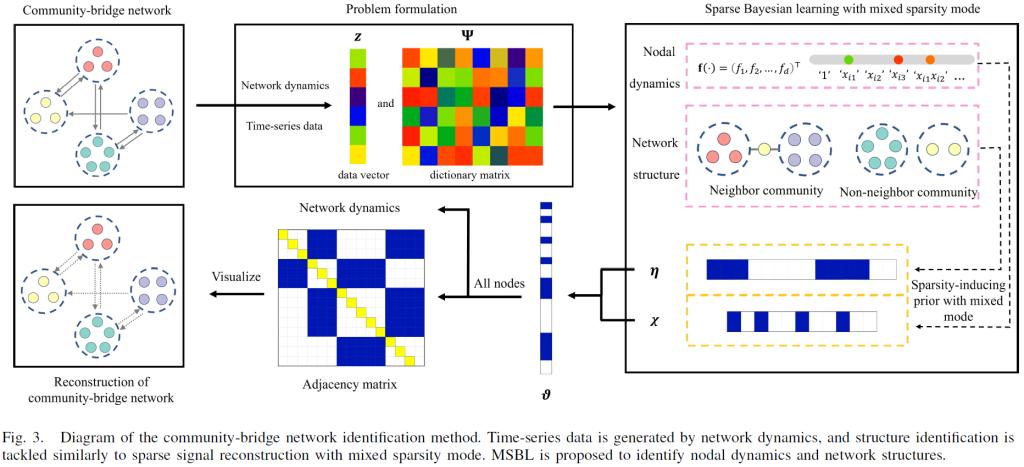
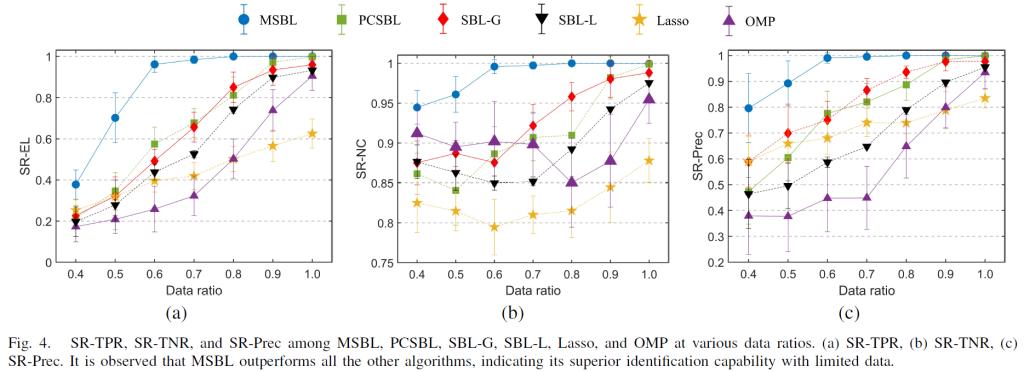