热烈祝贺课题组李李俊林博士一作论文Data-driven discovery of block-oriented nonlinear models using sparse null-subspace methods被影响因子大于10的国际控制论顶刊 IEEE Transactions on Cybernetics录用为长文!
Abstract: This paper develops an identification algorithm for nonlinear systems. Specifically, the nonlinear system identification problem is formulated as a sparse recovery problem of a homogeneous variant searching for the sparsest vector in the null-subspace. Augmented Lagrangian function is utilized to relax the nonconvex optimization. Thereafter, an algorithm based on alternating direction method and a regularization technique is proposed to solve the sparse recovery problem. The convergence of the proposed algorithm can be guaranteed through theoretical analysis. Moreover, by the proposed sparse identification method, redundant terms in nonlinear functional forms are removed and the computational efficiency is thus substantially enhanced. Numerical simulations are presented to verify the effectiveness and the superiority of the present algorithm.
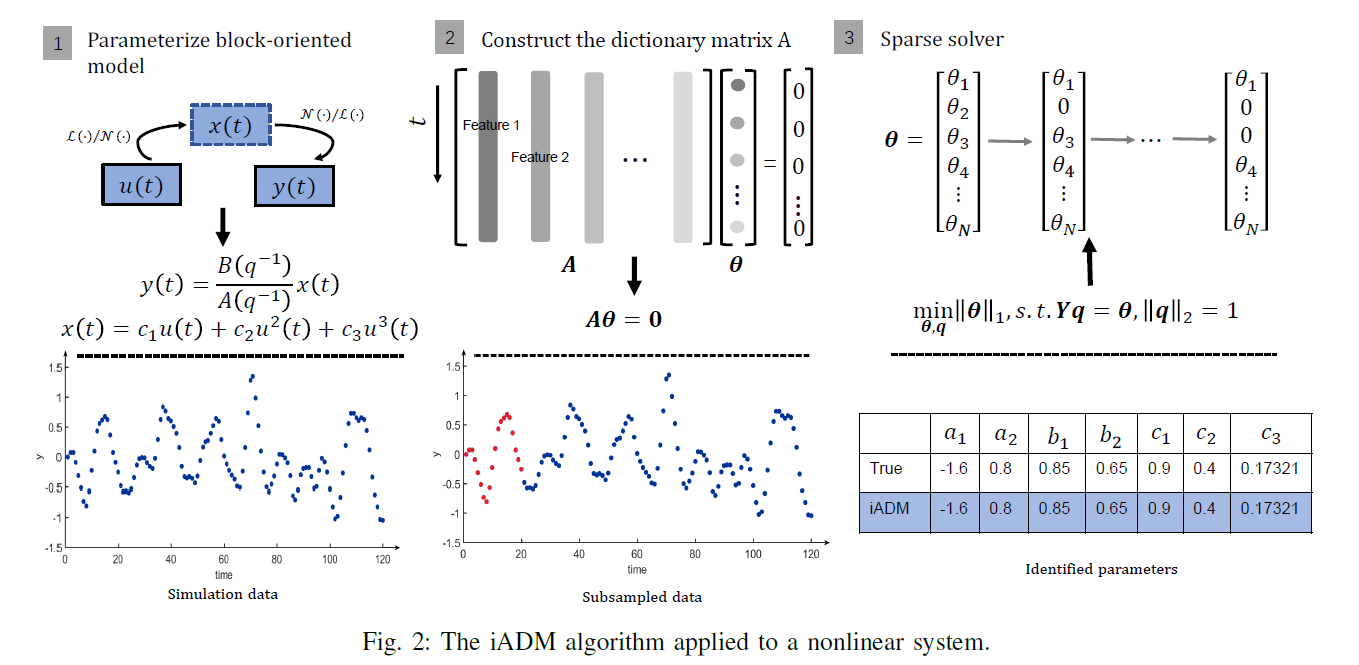
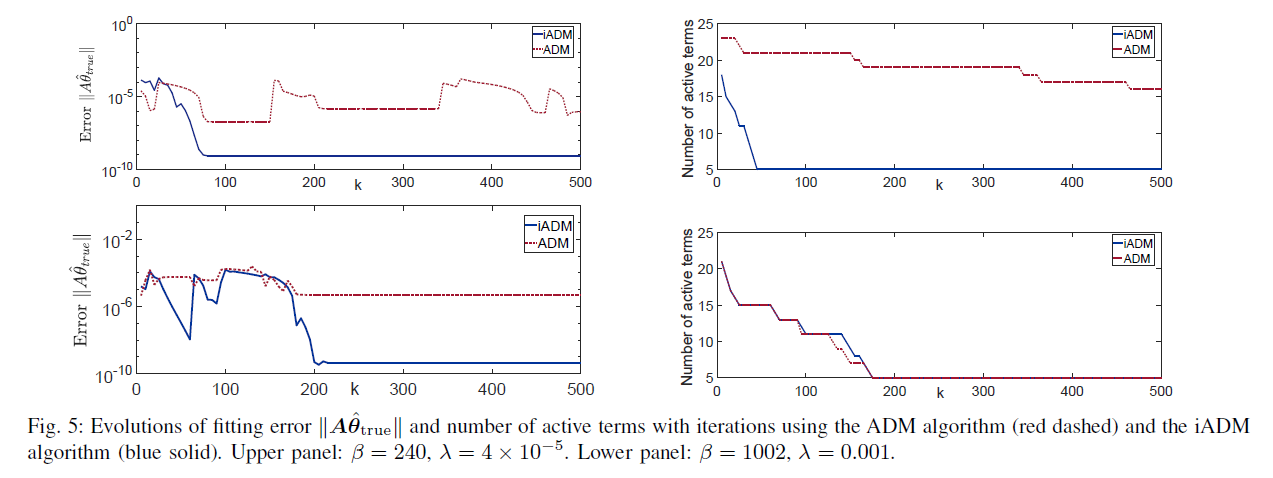