热烈祝贺周维博士的一作论文“An Efficient Sparse Bayesian Learning Algorithm Based on Gaussian Scale Mixtures”被国际人工神经网络顶刊IEEE TNNLS录用为长文
《An Efficient Sparse Bayesian Learning Algorithm Based on Gaussian Scale Mixtures》
Abstract: Sparse Bayesian learning (SBL) is a popular machine learning approach with a superior generalization capability due to the sparsity of its adopted model. However, it entails a matrix inversion at each iteration, hindering its practical applications with large-scale datasets. To overcome this bottleneck, we propose an efficient SBL algorithm with $\mathcal{O}(n^2)$ computational complexity per iteration based on a Gaussian scale mixture prior model. By specifying two different hyperpriors, the proposed efficient SBL algorithm can meet two different requirements; i.e., high efficiency and high sparsity. A surrogate function is introduced herein to approximate the posterior density of model parameters and thereby to avoid matrix inversions. Using a data-dependent term, a joint cost function with separate penalty terms is reformulated in a joint space of model parameters and hyperparameters. The resulting nonconvex optimization problem is solved by using a block coordinate descent method in a majorization-minimization framework. Finally, the results of extensive experiments for sparse signal recovery and sparse image reconstruction on benchmark problems are elaborated to substantiate the effectiveness and superiority of the proposed approach in terms of computational time and estimation error.
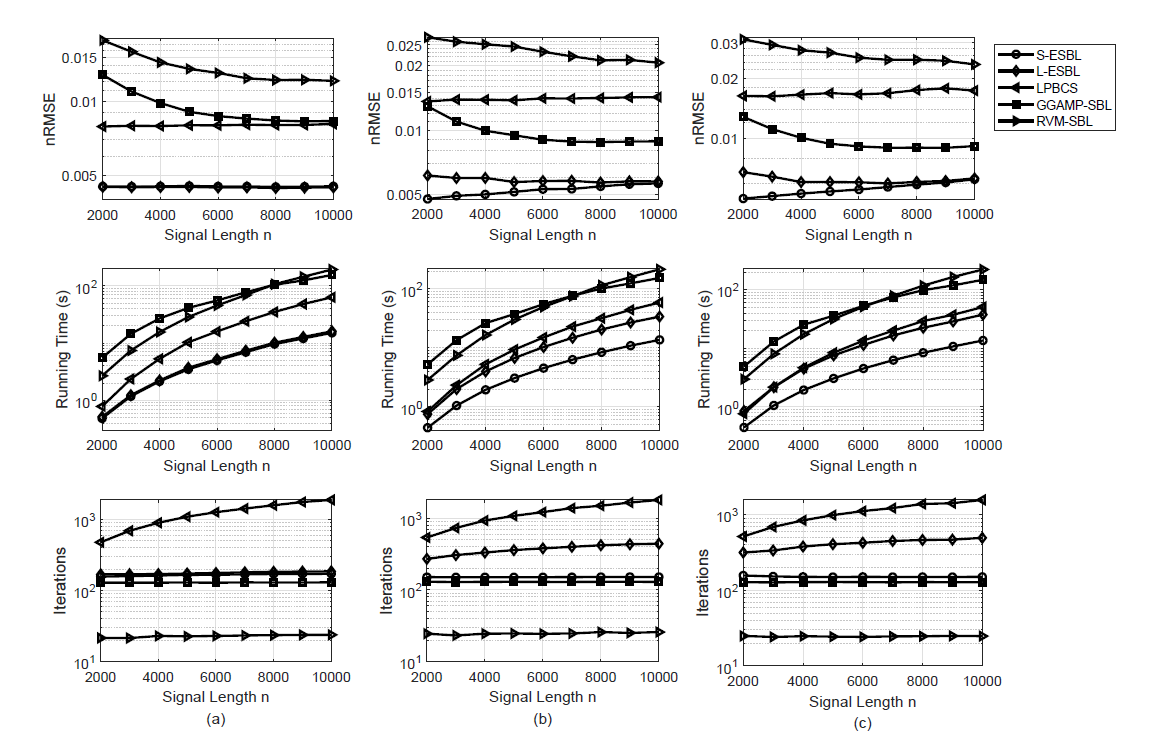
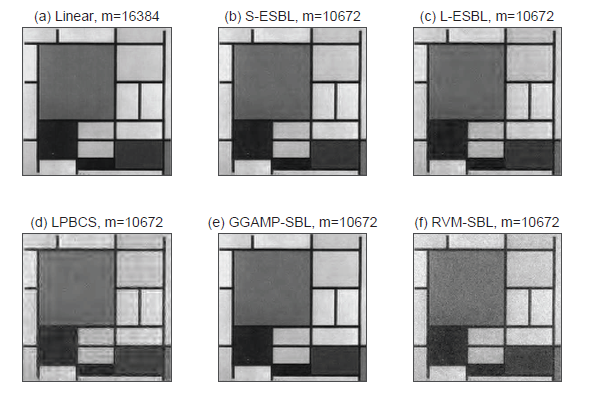