Abstract—Sparse representation has been widely applied to image classification, where the key issue is to extract a suitable discriminative dictionary. To this end, we propose a joint dictionary and classifier learning algorithm based on a parameterized Bayesian model. Therein, the Gaussian priors of a dictionary endow it the capability of discrimination and representation thanks to the restricted isometry property of the Gaussian random matrix. Moreover, we introduce a multivariate Gaussian prior for the sparse codes to achieve group-sparsity, thereby substantially improving the classification performance. Furthermore, the sparse codes are estimated by a group sparse Bayesian learning method, and the dictionary atoms are updated sequentially by maximizing a posterior. Moreover, to avoid manual parameter adjustment, the hyperparameters are optimized by an evidence maximization method. Accordingly, we develop a classification scheme via group sparse Bayesian learning. Finally, extensive experiments are conducted on six benchmark datasets of face classification, object recognition, handwritten recognition and scene categorization to substantiate the effectiveness and superiority of the proposed method. Index Terms—Dictionary learning, image classification, sparse representation, sparse Bayesian learning.

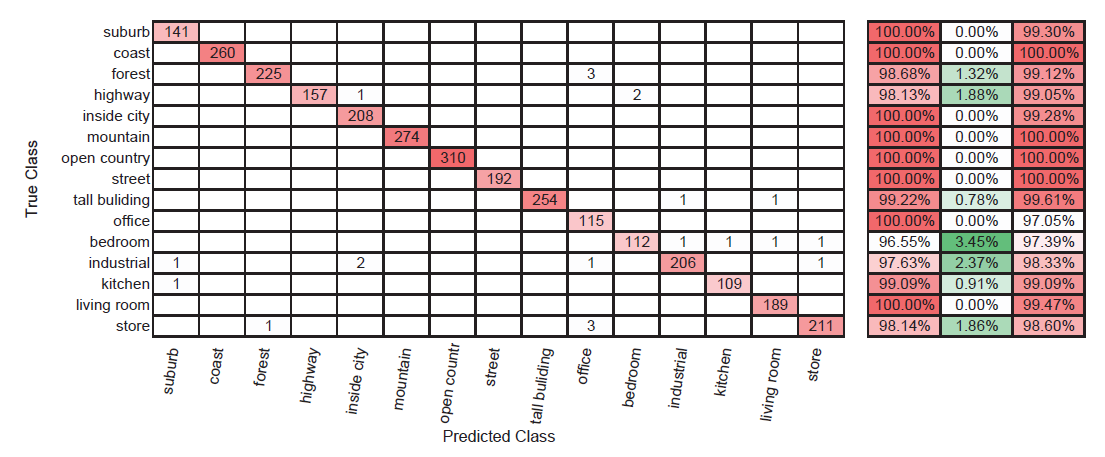