Summary
BackgroundMarket-applicable concurrent electrocardiogram (ECG) diagnosis for multiple heart abnormalities that covers a wide range of arrhythmias, with better-than-human accuracy, has not yet been developed. We therefore aimed to engineer a deep learning approach for the automated multilabel diagnosis of heart rhythm or conduction abnormalities by real-time ECG analysis.
MethodsWe used a dataset of ECGs (standard 10 s, 12-channel format) from adult patients (aged ≥18 years), with 21 distinct rhythm classes, including most types of heart rhythm or conduction abnormalities, for the diagnosis of arrhythmias at multilabel level. The ECGs were collected from three campuses of Tongji Hospital (Huazhong University of Science and Technology, Wuhan, China) and annotated by cardiologists. We used these datasets to develop a convolutional neural network approach to generate diagnoses of arrythmias. We collected a test dataset of ECGs from a new group of patients not included in the training dataset. The test dataset was annotated by consensus of a committee of board-certified, actively practicing cardiologists. To evaluate the performance of the model we assessed the F1 score and the area under the curve (AUC) of the receiver operating characteristic (ROC) curve, as well as quantifying sensitivity and specificity. To validate our results, findings for the test dataset were compared with diagnoses made by 53 ECG physicians working in cardiology departments who had a wide range of experience in ECG interpretation (range 0 to >12 years). An external public validation dataset of 962 ECGs from other hospitals was used to study generalisability of the diagnostic model.
FindingsOur training and validation dataset comprised 180 112 ECGs from 70 692 patients, collected between
Jan 1, 2012, and Apr 30, 2019. The test dataset comprised 828 ECGs corresponding to 828 new patients, recorded between Sept 11, 2012, and Aug 30, 2019. At the multilabel level, our deep learning approach to diagnosing heart abnormalities resulted in an exact match in 658 (80%) of 828 ECGs, exceeding the mean performance of physicians (552 [67%] for physicians with 0–6 years of experience; 571 [69%] for physicians with 7–12 years of experience; 621 [75%] for physicians with more than 12 years of experience). Our model had an overall mean F1 score of 0·887 compared with 0·789 for physicians with 0–6 years of experience, 0·815 for physicians with 7–12 years of experience, and 0·831 for physicians with more than 12 years of experience. The model had a mean AUC ROC score of 0·983 (95% CI 0·980–0·986), sensitivity of 0·867 (0·849–0·885) and specificity of 0·995 (0·994–0·996). Promising F1 scores were also obtained from the external public database using our proposed model without any model modifications (mean F1 scores of 0·845 in multilabel and 0·852 in single-label ECGs).
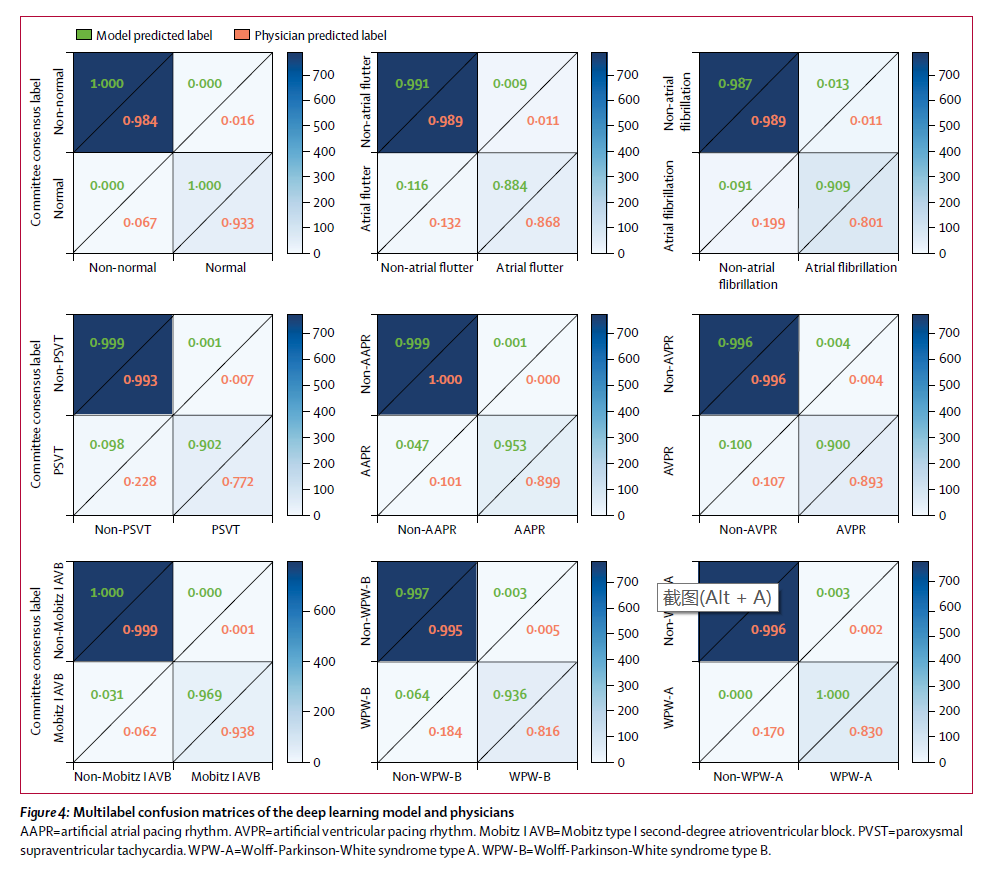