Links go to the original report:https://m.thepaper.cn/newsDetail_forward_6586157?from=singlemessage
The following is the report.
On March 17th local time,MedRXIV, a medical preprint platform, released a study titled "A Machine Learning-Based Model for Survival Prediction in Patients with Severe COVID-19Infection "(not peer reviewed). The study, conducted by 29 scientists, used the latest interpretable machine learning algorithms to uncover biomarkers that predict survival in patients with COVID-19 (COVID-19) and is expected to enhance early intervention for patients at high risk of COVID-19 to reduce mortality.
The research team is from Tongji Hospital, School of Artificial Intelligence and Automation of Huazhong University of Science and Technology, and School of Plant Science of Cambridge University. The corresponding authors of the paper are Ye Yuan, professor of the School of Artificial Intelligence and Automation, Huazhong University of Science and Technology, Hui Xu, director of the Department of Anesthesiology, Tongji Hospital, and Shusheng Li, director of the Department of Emergency (Critical Care) Medicine.
Blood sample data were collected from 404 patients with COVID-19 infection admitted to Tongji Hospital in Wuhan and analyzed retrospectively. Using machine learning tools, the team ultimately selected three biomarkers to predict individual patient survival with more than 90 percent accuracy:LDH (lactate dehydrogenase), lymphocytes and hs-CRP (hypersensitive C-reactive protein).
In particular,Only High LDH level can be used to distinguish the vast majority of cases requiring immediate medical attention. The researchers said the findings are consistent with current medical knowledge that high LDH levels are associated with tissue breakdown occurring in a variety of diseases, including lung diseases such as pneumonia.
At this stage,rapid, accurate and early clinical assessment of disease severity is essential. However, there are no established biomarkers as criteria to distinguish patients who require immediate medical attention.
In this study, the authors usedmost advancedmachine learning framework to show that these three biomarkers can accurately predict the severity of disease, thusgreatly reducing the pressure of clinical parameter monitoring and other associated medical burden.
Researchers developed a prognostic model based on XGBoost machine learning that predicted survival of COVID-19 patients with more than 90% accuracy using a patient's most recent blood sample;Using other blood samples, the prediction was 90 percent accurate.
This study proposes a simple and actionable formula for rapid detection, early intervention, and potentially reduced mortality in patients at high risk for COVID-19.
Study samples and model training
The researchers performed a categorization task, in which basic information, symptoms, blood samples, and laboratory test results (including liver function, kidney function, clotting function, electrolytes, and inflammatory factors) of patients with general, severe, and critical conditions were input, and corresponded them to clinical outcomes (survival or death) at the end of the testing period.
The study samples were collected from 404 patients in Tongji Hospital between January 10, 2020 and February 20, 2016. Of those 404 patients, 213 recovered and 191 died, the authors said, with the high mortality rate linked to Tongji Hospital's role as a designated hospital for treating the most severe cases. The researchers collected medical records using standard case report forms, which included epidemiological, demographic, clinical, medication, care, and mortality information.
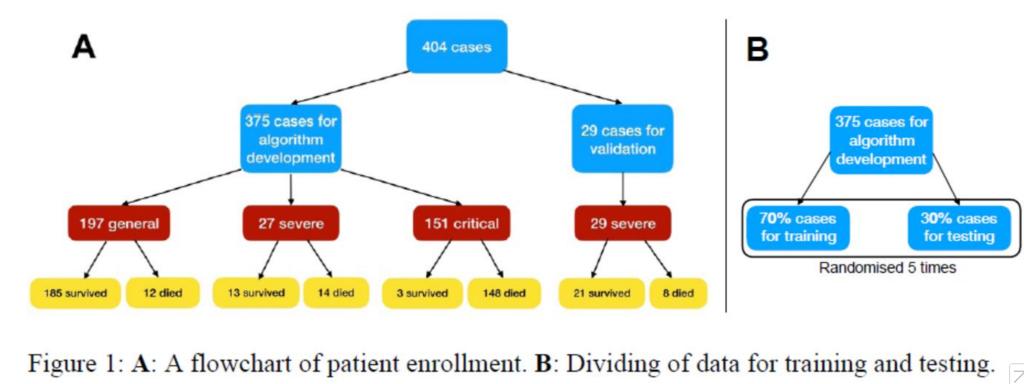
The researchers used the information of 375 patients for algorithm development and 29 cases as validation sets.
The patients' data were divided into training set, test, and additional validation set.The training and testing set included a total of 375 patients, while the validation set included 29 patients.The number of samples in the training and test sets was set in a ratio of 7:3, and cross-validation was performed for 5 times.
Patients in theadditional validation setare all severely ill because they are the least predictable in terms of clinical outcomes. In terms of clinical symptoms, fever was the most common initial symptom (49.9%), followed by cough (13.9%), fatigue (3.7%) and dyspnea (2.1%). The age distribution of 375 patients was 58.83±16.46 years, with males accounting for 58.7%.Among the patients, 37.9% were residents of Wuhan, 6.4% were family cluster cases, and 1.9% were medical workers.
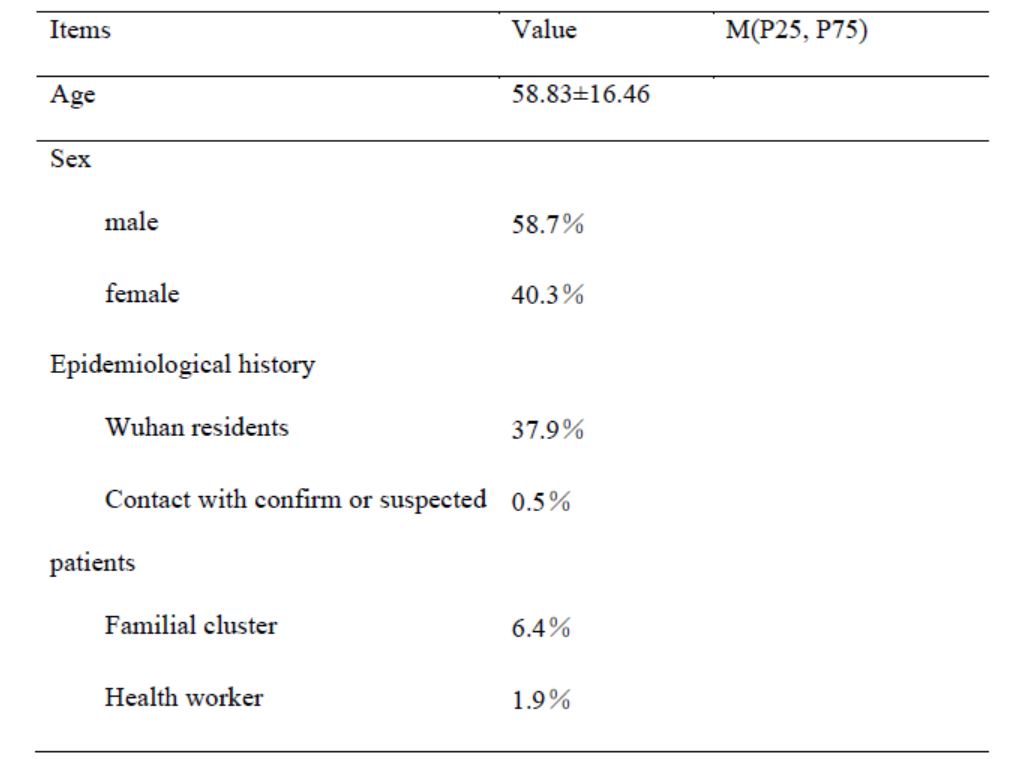
The characteristics of age, sex and epidemic history of the sample patients were analyzed
Although most patients with multiple blood samples collected in hospital, but the model using only the training and test samples from patients with a recent record as input, in order to get the key biomarkers to evaluate the severity of disease biomarkers, distinguish between patients need immediate medical assistance and accurate matching corresponding function of each label.
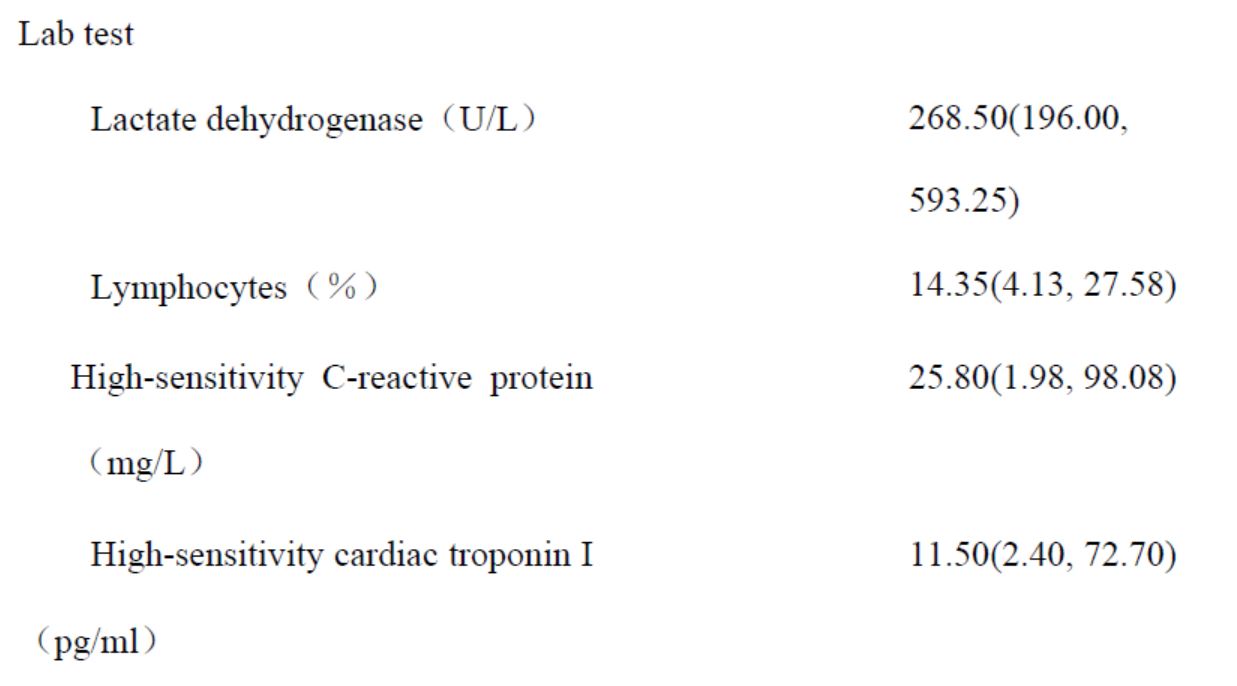
The median value of the three biomarkers and the 25 and 75 percentile values of the patients .
The clinical features most associated with mortality risk
The researchers used a classifier called XGBoost, a high-performance machine learning algorithm that has great interpretability due to its tree-based recursive decision system,as a predictor model.The output of the model corresponded to patient survival, with the researchers classifying patients who survived as category 0 and patients who died as category 1.
The reason why the researchers did not use the black-box modelling strategy is that its internal modeling mechanisms are often difficult to explain. In XGBoost, the importance of each individual feature is determined by its cumulative usage at each decision step in the tree. In this way, a metric can be derived to describe the relative importance of each feature, which is particularly valuable for evaluating the most differentiated features in model outcomes, especially when the study is related to clinical medical parameters.
To assess markers for the risk of death occurring, the researchers assessed the contribution of each patient's parameters to the algorithm's decision through a feature selection process. XGBoost ranks functions according to their importance, andthe algorithm selects the top three clinical features: LDH, lymphocytes, and hs-CRP,and therefore, they are set as critical features.
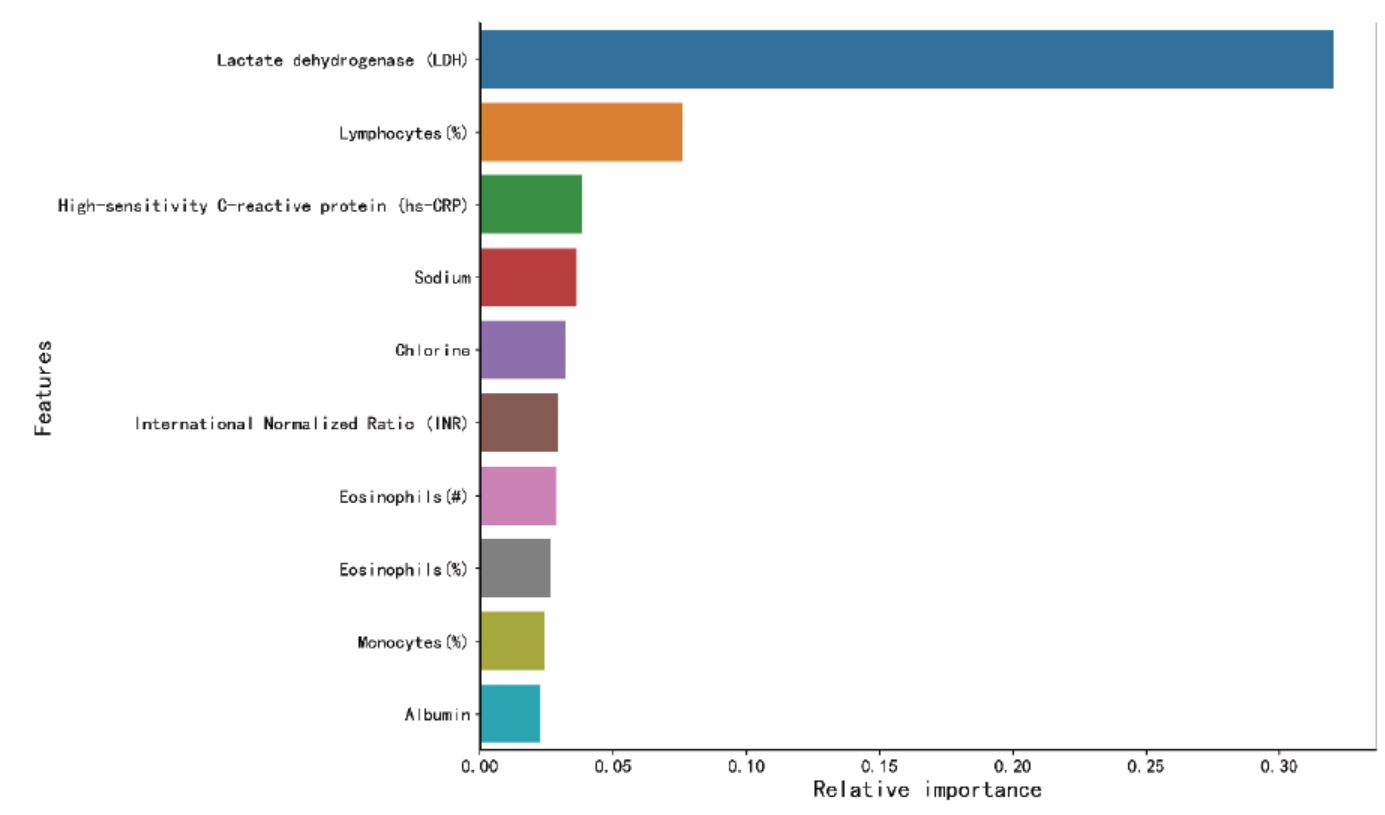
The researchers ranked the top 10 key clinical features according to their importance in the multi-tree XGBoost algorithm, with LDH, lymphocytes and hs-CRP ranking in the top three.
The results show thatthe model can accurately predict the outcome of patients, regardless of the initial diagnosis on admission.
In addition, the performance of additional validation set was similar to that of training and test sets, indicating that the model captured key biomarkers related to patient survival.At the same time, the algorithm results further emphasize the importance of LDH as a key biomarker for patient survival.
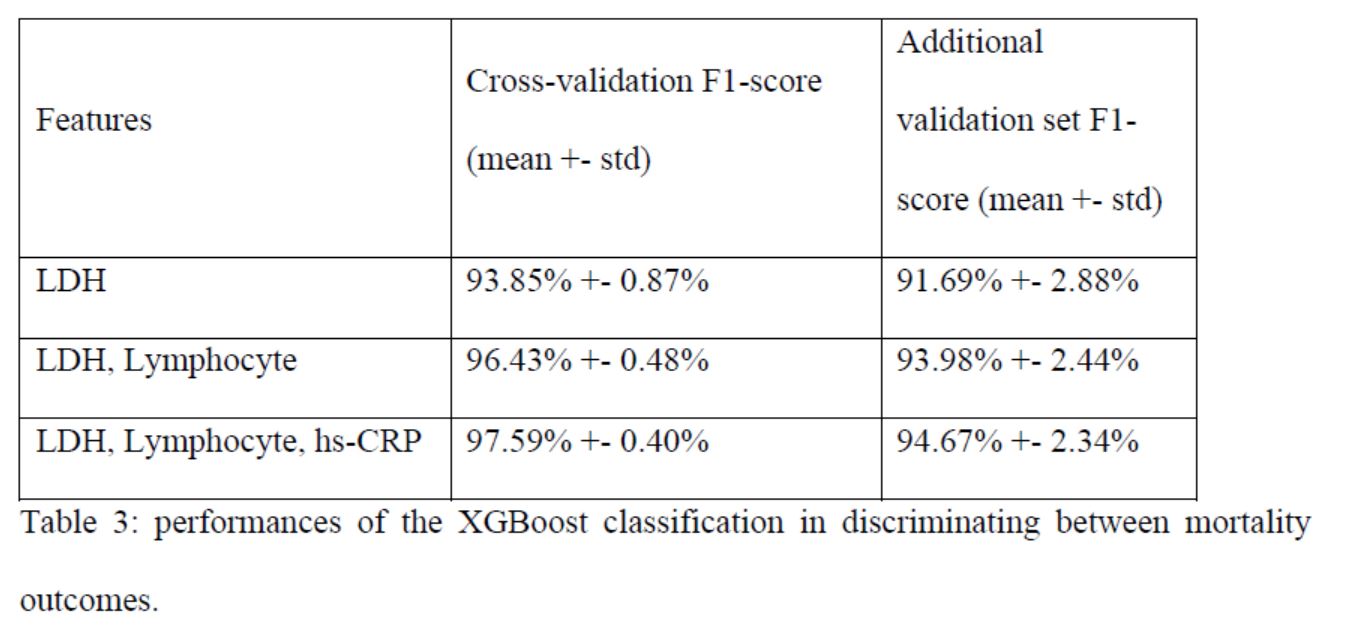
For the three key features of training/test split and model performance of additional validation set, F1-score is the harmonic average of the algorithm accuracy rate and recall rate, with a maximum of 1 and a minimum of 0.
Based on the findings about importance of LDH, lymphocytes, and hs-CRP, the researchers further constructed a simplified and clinically applicable decision model, the single decision tree.
Because a total of 24 patients had incomplete measurements for at least one of the three major biomarkers, the researchers used the remaining 351 patients to identify the single-tree XGBoost model.
In short,the researchers chose the best-performing tree in the model, using three key features and their thresholds to predict whether a patient would die or survive.
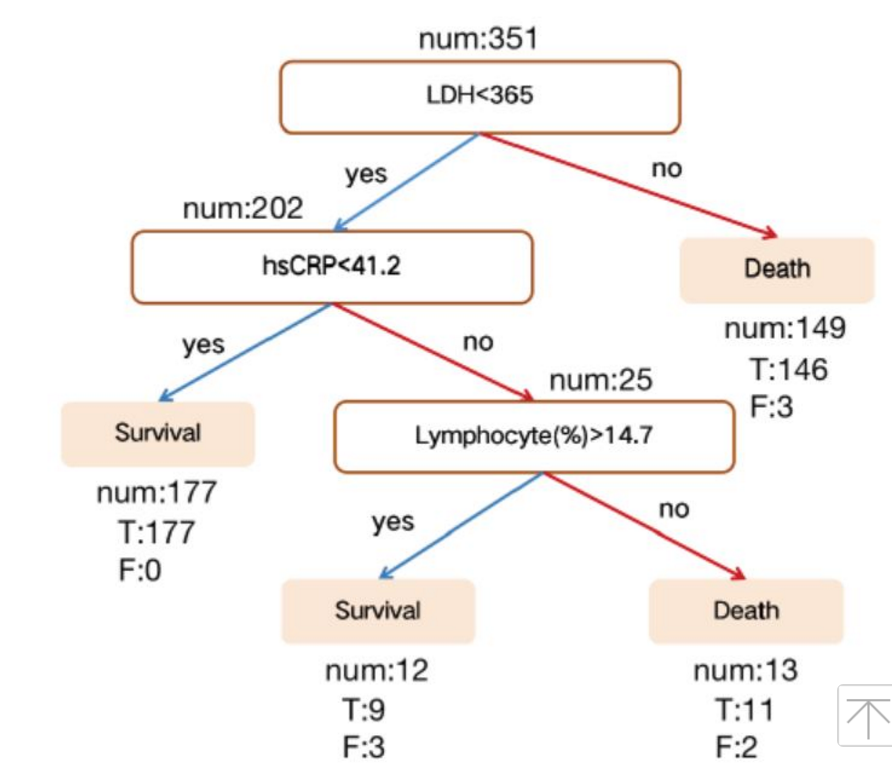
Select the best-performing tree and its accuracy based on the test data set.
This model shows 100% accuracy for death and 90% accuracy for survival. Overall, both the multi-tree and single-tree XGBoost models consistently scored higher than 0.90 for survival and death prediction accuracy, macroscopic and weighted averages.
Finally, most patients receive multiple blood samples during their stay in the hospital.The researchers then validated the model with the results of thousands of additional blood tests andfound that the prediction was 90 percent accurate. In addition, the results further demonstrate that the model can be applied to any blood sample, regardless of the patient's clinical outcome.
Early identification of high-risk patients and rapid prioritization.
The researchers said implications of this study are twofold.Firstly, general conventional studies only "provide a range of risk factors," this model provides a simple and intuitive clinical test that can accurately and quickly quantify the risk of death.
If doctors know ahead of time that certain treatments may lead to poor outcomes for some patients, they may be able to adopt different approaches before the symptoms become more severe.The goal of using this model is to identify high-risk patients before irreversible lesions occur.
Secondly, any hospital can easily collect information on patients' three key indicators: LDH (lactate dehydrogenase), lymphocytes, and hs-CRP (hypersensitive C-reactive protein). This simple model can help prioritize quickly patients in crowded hospitals where medical resources are scarce.
Increased LDH levels in patients can reflect tissue or cell damage and are considered a common sign of tissue or cell damage.Serum LDH has been identified as an important biomarker for the severity of idiopathic pulmonary fibrosis (IPF).
The increase in LDH is significant in patients with severe pulmonary interstitial disease and is one of the most important prognostic indicators of lung injury in patients.Thus, in patients with severe COVID-19, elevated LDH levels indicate increased severity of lung injury.
The team noted higher serum hs-CRP values may also be used to predict the risk of death in patients with severe COVID-19.An increase in hs-CRP is an important marker of poor prognosis in patients with ARDS, reflecting the ongoing state of inflammation.
Notably, the results of this persistent inflammatory response can be seen in the autopsy of the COVID-19 deceased, with numerous grayish-white lesions in the lungs and a large amount of mucous secretions spillage from the alveoli in the tissue sections.
Finally,the results also suggest that lymphocytes may serve as potential therapeutic targets, this hypothesis supported by clinical findings.In addition, previous researchers including Bin Cao's team, director of the Department of Inhalation at the China-Japan Friendship Hospital in Beijing, have shown that lymphoenia is a common feature of COVID-19 patients and may be a key factor associated with disease severity and mortality.
In the same way that alveolar penetration and antigen-presenting cells (APC) were damaged in SARS and MERS patients, the damaged alveolar epithelial cells in COVID-19 patients induced lymphocyte infiltration, leading to persistent lymphocytopenia.
A previous patient biopsy study showed that the number of CD4 and CD8 T cells in peripheral blood was significantly reduced, while their state was overactivated.In addition, some studies have shown that lymphocytopenia is mainly associated with a decrease in CD4 and CD8 T cells.Therefore, lymphocytes may play an obvious role in COVID-19, which warrants further investigation.
The authors said this study also has limitations.Firstly, since this machine learning approach is purely data-driven, the model may be different if you start with a different data set.
In addition, although the authors had more than 80 clinical measurements, in order to avoid overfitting, the modeling principle used by the team was a trade-off between minimum number of clinical measurements and good predictive power, so there might be a lack of clinical measurements.
Finally, the study balances model's interpretability with greater accuracy.Although clinical settings tend to use interpretable models, the black-box model may be more accurate, but at the same time the decision risk is higher.
From a technical perspective, the authors believe this work could help use machine learning methods predict and diagnose COVID-19 cases in an ongoing global outbreak.